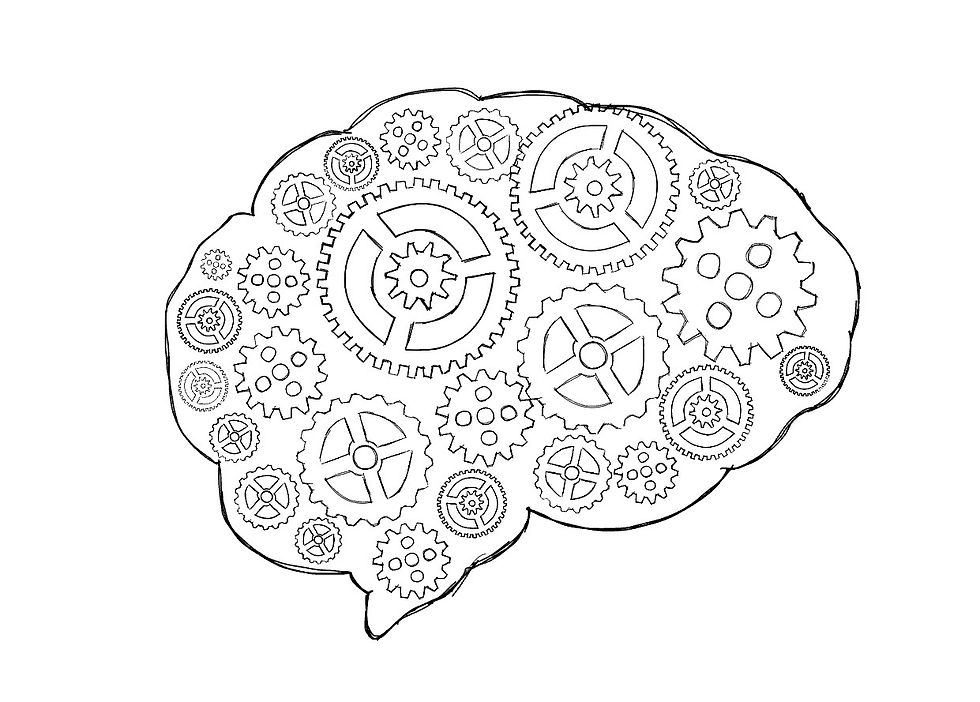
Introduction:
Machine Learning (ML) is a pivotal subset of Artificial Intelligence (AI) that has reshaped numerous industries. In this in-depth blog post, we will explore the origins of machine learning, delve into its technical aspects, and discuss its wide variety of applications. From image recognition and computer vision to remote sensing and self-driving cars, ML has made significant strides, driven by the advancements in AI. We will also address concerns and fears surrounding machine learning and AI while looking towards the future and exploring potential theoretical applications.
Unveiling the Origins of Machine Learning:
Machine Learning has its roots in computer science and early AI research. In the 1950s and 1960s, researchers focused on developing neural networks and algorithms for pattern recognition. These early endeavors laid the foundation for ML, which aims to enable machines to learn and improve from experience without explicit programming. Notable pioneers include Frank Rosenblatt with his perceptron model and Arthur Samuel with his work on game-playing algorithms.
The concepts of ML gained traction as computing power increased and the availability of vast amounts of data grew. In the 1990s, the advent of powerful algorithms such as support vector machines (SVMs) and decision trees further advanced the field. This laid the groundwork for the explosion of ML applications in recent years.
Understanding the Technical Aspects of Machine Learning:
Machine Learning encompasses a range of techniques and algorithms that empower systems to learn from data. Let's delve into some key technical aspects:
a) Supervised Learning:
Supervised learning involves training ML models using labeled data, where each data point is associated with a known output or label. Notable algorithms in this domain include support vector machines (SVMs), decision trees, random forests, and deep learning architectures like convolutional neural networks (CNNs) and recurrent neural networks (RNNs).
b) Unsupervised Learning:
Unsupervised learning focuses on training models on unlabeled data, allowing them to identify patterns or relationships without prior knowledge of the output. Popular unsupervised learning techniques include clustering algorithms like k-means, hierarchical clustering, and Gaussian mixture models. Dimensionality reduction methods such as principal component analysis (PCA) and t-distributed stochastic neighbor embedding (t-SNE) help to uncover underlying structures in high-dimensional data.
c) Reinforcement Learning:
Reinforcement learning revolves around training agents to interact with an environment and learn optimal actions through trial and error. The agent receives feedback in the form of rewards or penalties, allowing it to improve its decision-making process over time. Notable algorithms in reinforcement learning include Q-learning, deep Q-networks (DQNs), and policy gradient methods like Proximal Policy Optimization (PPO) and Trust Region Policy Optimization (TRPO).
Wide Variety of Machine Learning Applications:
Machine Learning has found applications across diverse domains. Let's explore specific examples that showcase its impact:
a) Image Recognition and Computer Vision:
Companies like Google, Facebook, and Microsoft have harnessed the power of ML to advance image recognition and computer vision tasks. Facial recognition algorithms have become highly accurate, enabling applications in security systems, user authentication, and social media tagging. Object detection algorithms can identify and track multiple objects in real-time, facilitating applications in autonomous vehicles, surveillance systems, and robotics.
b) Remote Sensing and Earth Observation:
Satellite imagery and remote sensing data are harnessed with ML techniques to monitor land cover changes, predict weather patterns, and aid in disaster management. Companies like Planet Labs and DigitalGlobe utilize ML algorithms for high-resolution image analysis and classification. This enables applications such as urban planning, agriculture monitoring, and climate change research.
c) Self-Driving Cars and Autonomous Systems:
Machine Learning plays a critical role in developing self-driving cars. Companies like Tesla, Waymo (Google), and Uber are at the forefront of this technology. ML algorithms process data from sensors such as cameras, lidar, and radar to recognize and track objects, plan routes, and make real-time decisions, enhancing road safety and transforming transportation. Similar ML techniques are applied to autonomous robots used in warehouses, delivery services, and industrial automation.
d) Natural Language Processing (NLP) and Language Translation:
NLP, powered by ML, enables machines to understand and generate human language. Companies like OpenAI, IBM, and Amazon leverage ML algorithms for tasks such as sentiment analysis, language translation, chatbots, and voice assistants like Amazon Alexa and Google Assistant. These advancements have improved the accessibility and usability of information and services across different languages and cultures.
e) Healthcare and Medical Applications:
Machine Learning has the potential to revolutionize healthcare. Companies and research institutions employ ML algorithms to aid in disease diagnosis, personalized treatment plans, drug discovery, genomics research, and medical imaging analysis. For example, ML algorithms can analyze medical images to detect abnormalities, predict disease progression, and assist radiologists in making accurate diagnoses. Startups like Zebra Medical Vision and Aidoc have developed ML-based solutions for medical imaging analysis.
f) Financial Services and Fraud Detection:
ML algorithms are employed in the financial sector for tasks like fraud detection, credit scoring, algorithmic trading, and risk assessment. Companies like PayPal, Capital One, and Visa utilize ML to analyze vast amounts of financial data and identify anomalies, patterns, and trends. Fraud detection algorithms can detect suspicious activities in real-time, helping to prevent fraudulent transactions and protect financial systems.
Addressing Concerns and Looking to the Future:
While the progress in machine learning and AI is commendable, it also raises concerns about ethics, privacy, and job displacement. There are legitimate fears regarding biases in algorithms, potential security breaches, and the impact on the job market. It is crucial for researchers, policymakers, and industry leaders to address these concerns and develop frameworks for responsible AI.
One potential approach to addressing these concerns is the development of explainable AI, where ML algorithms provide interpretable results and justifications for their decisions. Efforts are also being made to ensure data privacy and mitigate biases in ML models through rigorous evaluation and diverse training datasets.
Looking to the future, the potential applications of machine learning are vast and exciting. ML algorithms have the potential to assist in climate modeling, drug discovery, personalized education, smart cities, and personalized medicine. For example, ML techniques can contribute to predicting and mitigating the impact of climate change by analyzing environmental data and simulating scenarios. ML-powered virtual tutors and personalized learning systems can adapt to individual student needs, improving education outcomes.
Furthermore, ML techniques can aid in the discovery of new drugs by analyzing vast amounts of genomic and chemical data, accelerating the drug development process. Smart city initiatives can leverage ML algorithms to optimize traffic flow, energy usage, waste management, and public safety, enhancing the quality of urban life.
Conclusion:
Machine Learning has emerged as a vital component of Artificial Intelligence, revolutionizing various industries. Its origins can be traced back to early AI research, and it has since grown into a powerful tool with diverse applications. From image recognition and remote sensing to self-driving cars and healthcare, ML has transformed industries and driven innovation.
As the field progresses, it is essential to address concerns surrounding ethics, biases, and job displacement. Responsible AI development, transparent algorithms, and robust regulations are key to mitigating these concerns.
By fostering responsible AI development and leveraging the potential of ML in emerging technologies like blockchain, augmented reality, and quantum computing, we can unlock new possibilities and shape a future that harnesses the true potential of machine learning and artificial intelligence. While challenges lie ahead, it is through ethical practices, continued research, and collaboration that we can harness the full potential of machine learning, ensuring a positive and impactful future for AI and society as a whole.
Comments